2-2 明るすぎたり暗すぎたりするときに有効「γ補正」
img_src = cv2.imread("data/Mandrill.png")
#ルックアップテーブルの設定
gamma = 0.8
look_up_table = np.zeros((256, 1), dtype = 'uint8')
for i in range(256):
look_up_table[i][0] = 255 * pow(float(i) / 255, 1.0 / gamma)
img_dst = cv2.LUT(img_src, look_up_table)
#描画する
cv2.imshow("img_src",img_src)
cv2.imshow("img_dst",img_dst)
cv2.waitKey(0)
cv2.destroyAllWindows()
#ヒストグラム
fig = plt.figure()
ax1 = fig.add_subplot(311) #総行数,総列数、サブプロット番号
ax2 = fig.add_subplot(312)
ax3 = fig.add_subplot(313)
color_list = ["blue","green","red"]
for i,j in enumerate(color_list):
hist = cv2.calcHist([img_src],[i],None,[256],[0,256])
ax1.plot(hist,color = j)
for i,j in enumerate(color_list):
hist = cv2.calcHist([img_dst],[i],None,[256],[0,256])
ax2.plot(hist,color = j)
x = [x for x in range(256)]
for gamma in range(1,30,2): #gamma = 0.1,0.2,0.5 ... 2.9
lut = [255 * pow(float(x) / 255, 1.0 / (gamma/10)) for x in range(256)]
ax3.plot(x,lut)
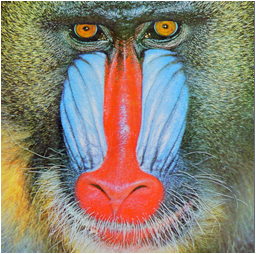
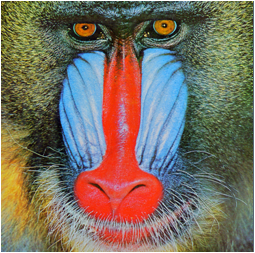
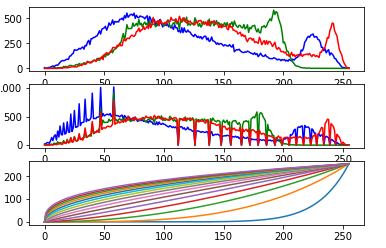