2-6 もっとも優れたコントラスト改善法のひとつ「ヒストグラム平坦化」
かなり時間がかかったpythonには向いていない
img_src = cv2.imread("data/inpu_img_2-6.bmp")
#画像の大きさ
eight = img_src.shape[0] #639
width = img_src.shape[1] #960
#ヒストグラムの作成
color_list = ["gray"]
for i,j in enumerate(color_list):
hist = cv2.calcHist([img_src],[i],None,[256],[0,256])
#ヒストグラムの平均値
sum = 0
ehn = np.empty_like(hist)
for i in range(256):
sum = sum + hist[i]
ehn[i] = sum * 255/(eight*width)
img_dst = np.zeros_like(img_src)
for i in range(256):
for y in range(eight):
for x in range(width):
if img_src[y,x,0] == i:
img_dst [y,x,0] = ehn[i]
img_dst [y,x,1] = ehn[i]
img_dst [y,x,2] = ehn[i]
cv2.imshow("img_src",img_src)
cv2.imshow("img_dst",img_dst)
cv2.waitKey(0)
cv2.destroyAllWindows()
#ヒストグラム
fig = plt.figure()
ax1 = fig.add_subplot(311) #総行数,総列数、サブプロット番号
ax2 = fig.add_subplot(312)
ax1.set_ylim(0,30000)
ax2.set_ylim(0,30000)
color_list = ["gray"]
for i,j in enumerate(color_list):
hist = cv2.calcHist([img_src],[i],None,[256],[0,256])
ax1.plot(hist,color = j)
for i,j in enumerate(color_list):
hist = cv2.calcHist([img_dst],[i],None,[256],[0,256])
ax2.plot(hist,color = j)
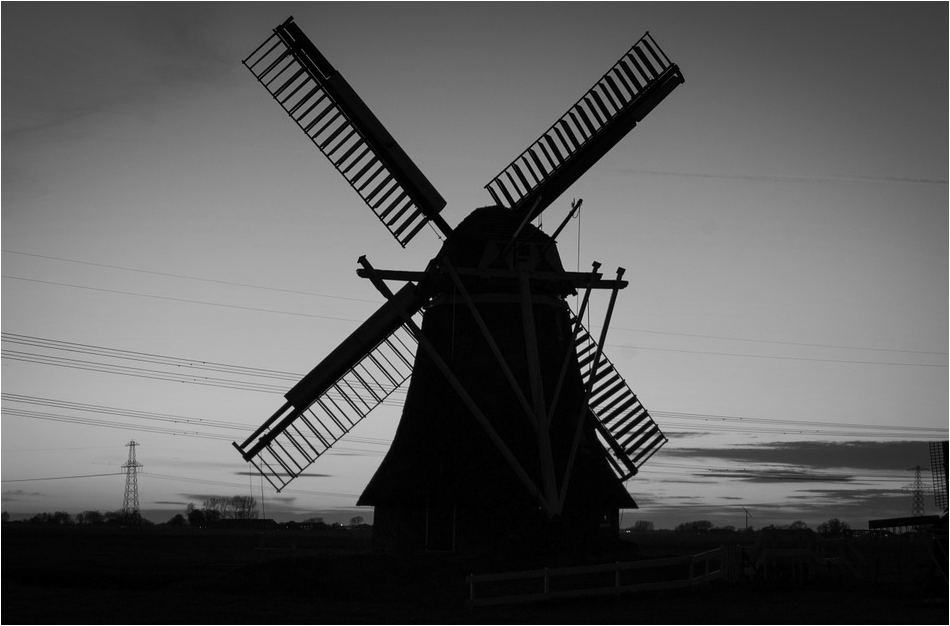
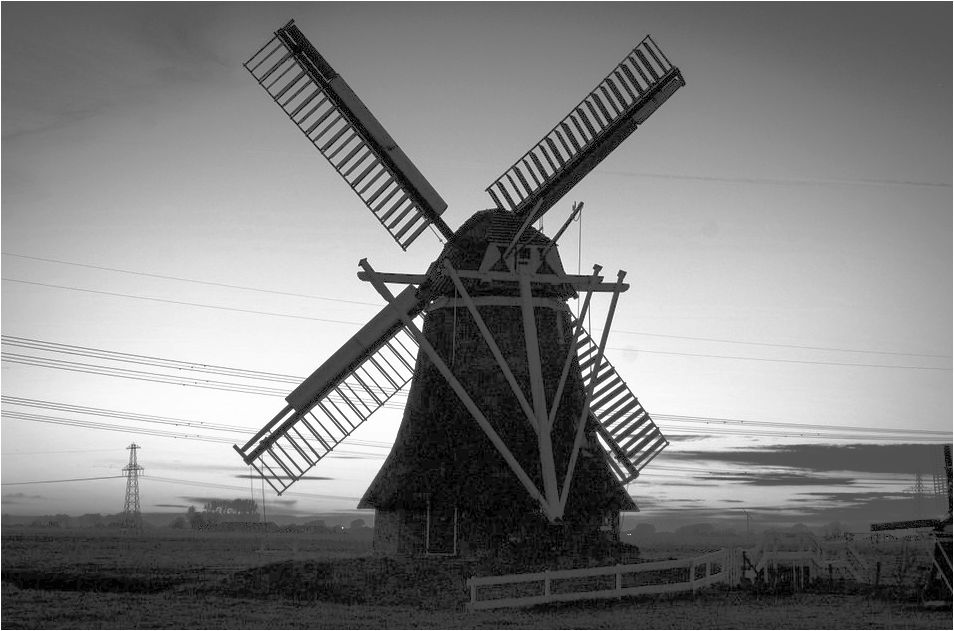
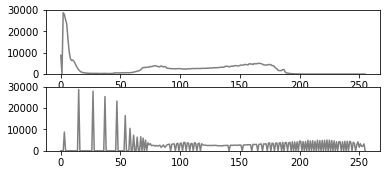